Over the next two days, the UK government is hosting an AI Safety Summit focused on “the safe and responsible development of frontier AI”. They requested that seven companies (Amazon, Anthropic, DeepMind, Inflection, Meta, Microsoft, and OpenAI) “outline their...
Over the next two days, the UK government is hosting an AI Safety Summit focused on “the safe and responsible development of frontier AI”. They requested that seven companies (Amazon, Anthropic, DeepMind, Inflection, Meta, Microsoft, and OpenAI) “outline their AI Safety Policies across nine areas of AI Safety”.
Below, I’ll give my thoughts on the nine areas the UK government described; I’ll note key priorities that I don’t think are addressed by company-side policy at all; and I’ll say a few words (with input from Matthew Gray, whose discussions here I’ve found valuable) about the individual companies’ AI Safety Policies.1
My overall take on the UK government’s asks is: most of these are fine asks; some things are glaringly missing, like independent risk assessments.
My overall take on the labs’ policies is: none are close to adequate, but some are importantly better than others, and most of the organizations are doing better than sheer denial of the primary risks.
1. Thoughts on the AI Safety Policy categories
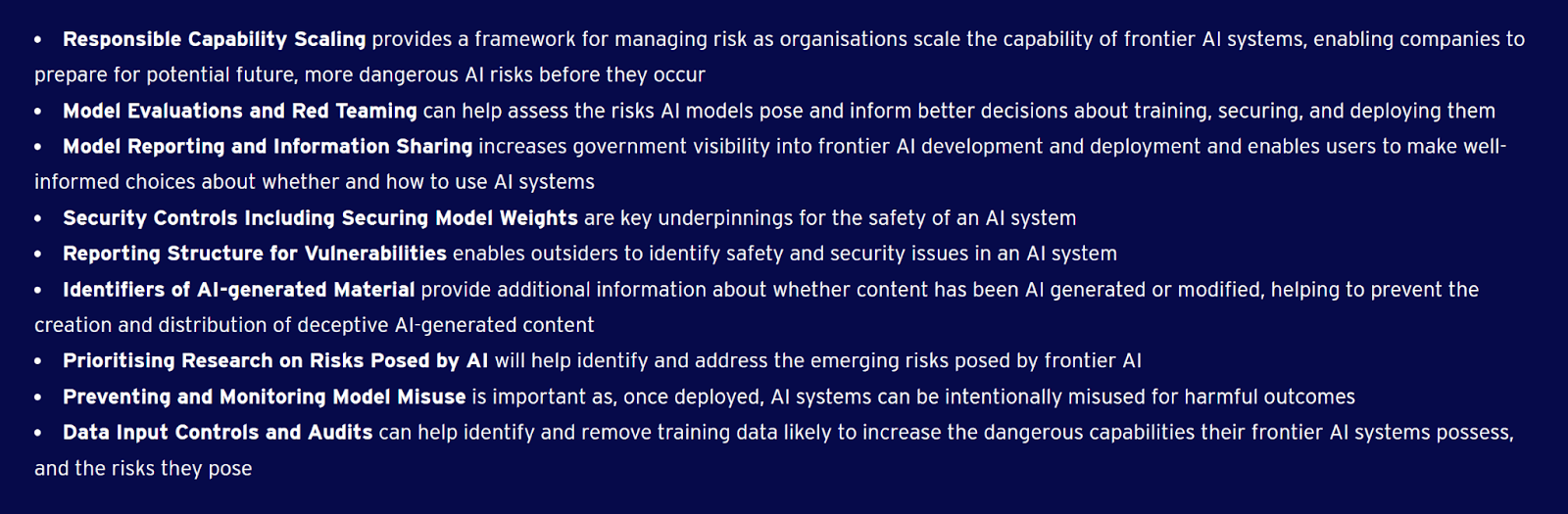
Responsible Capability Scaling provides a framework for managing risk as organisations scale the capability of frontier AI systems, enabling companies to prepare for potential future, more dangerous AI risks before they occur
There is no responsible scaling of frontier AI systems right now — any technical efforts that move us closer to smarter-than-human AI come with an unacceptable level of risk.2
That said, it’s good for companies to start noting conditions under which they’d pause, as a first step towards the sane don’t-advance-toward-the-precipice-at-all policy.
In the current regime, I think our situation would look a lot less dire if developers were saying “we won’t scale capabilities or computational resources further unless we really need to, and we consider the following to be indicators that we really need to: [X]”. The reverse situation that we’re currently in, where the default is for developers to scale up to stronger systems and where the very most conscientious labs give vague conditions under which they’ll stop scaling, seems like a clear recipe for disaster. (Albeit a more dignified disaster than the one where they scale recklessly without ever acknowledging the possible issues with that!)
Model Evaluations and Red Teaming can help assess the risks AI models pose and inform better decisions about training, securing, and deploying them
You’ll need to evaluate more than just foundation models, but evaluation doesn’t hurt. There’s an important question about what to do when the evals trigger, but evals are at least harmless, and can be actively useful in the right circumstance.
Red teaming is reasonable. Keep in mind that red teamers may need privacy and discretion in order to properly do their work.
Red teaming would obviously be essential and a central plank of the technical work if we were in a position to solve the alignment problem and safely wield (at least some) powerful AGI systems.
We mostly aren’t in that position: there’s some faint hope that alignment could turn out to be tractable in the coming decades, but I think the main target we should be shooting for is an indefinite pause on progress toward AGI, and a redirection of efforts away from AGI and alignment and toward other technological avenues for improving the world. The main value I see from evals and red teaming at this point is that they might make it obvious sooner that a shutdown is necessary, and they might otherwise slow down the AGI race to some extent.
Model Reporting and Information Sharing increases government visibility into frontier AI development and deployment and enables users to make well-informed choices about whether and how to use AI systems
This seems clearly good, given the background views I outlined above. I advise building such infrastructure.
Security Controls Including Securing Model Weights are key underpinnings for the safety of an AI system
This seems good; even better would be to make it super explicit that the Earth can’t survive open-sourcing model weights indefinitely. At some point (possibly in a few decades, possibly next year), AIs will be capable enough that open-sourcing those capabilities effectively guarantees human extinction.
And publishing algorithmic ideas, open-sourcing model weights, etc. even today causes more harm than good. Publishing increases the number of actors in a way that makes it harder to mitigate race dynamics and harder to slow down; when a dangerous insight is privately found, it makes it easier for others to reconstruct the dangerous insight by following the public trail of results prior to closure; and publishing contributes to a general culture of reflexively sharing insights without considering their likely long-term consequences.
Reporting Structure for Vulnerabilities enables outsiders to identify safety and security issues in an AI system
Sure; this idea doesn’t hurt.
Identifiers of AI-generated Material provide additional information about whether content has been AI generated or modified, helping to prevent the creation and distribution of deceptive AI-generated content
This seems like good infrastructure to build out, especially insofar as you’re actually building the capacity to track down violations. The capacity to know everyone who’s building AIs and make sure that they’re following basic precautions is the key infrastructure here that might help you further down the line with bigger issues.
Prioritising Research on Risks Posed by AI will help identify and address the emerging risks posed by frontier AI
This section sounds like it covers important things, but also sounds somewhat off-key to me.
For one thing, “identify emerging risks” sounds to me like it involves people waxing philosophical about AI. People have been doing plenty of that for a long time; doing more of this on the margin mostly seems unhelpful to me, as it adds noise and doesn’t address civilization’s big bottlenecks regarding AGI.
For another thing, the “address the emerging risks” sounds to me like it visualizes a world where labs keep an eye on their LLMs and watch for risky behavior, which they then address before proceeding. Whereas it seems pretty likely to me that anyone paying careful attention will eventually realize that the whole modern AI paradigm does not scale safely to superintelligence, and that wildly different (and, e.g., significantly more effable and transparent) paradigms are needed.
If that’s the world we live in, “identify and address the emerging risks” doesn’t sound quite like what we want, as opposed to something more like “prioritizing technical AI alignment research”, which phrasing leaves more of a door open to realizing that an entire development avenue needs abandoning, if humanity is to survive this.
(Note that this is a critique of what the UK government asked for, not necessarily a critique of what the AI companies provided.)3
Preventing and Monitoring Model Misuse is important as, once deployed, AI systems can be intentionally misused for harmful outcomes
Setting up monitoring infrastructure seems reasonable. I doubt it serves as much of a defense against the existential risks, but it’s nice to have.
Data Input Controls and Audits can help identify and remove training data likely to increase the dangerous capabilities their frontier AI systems possess, and the risks they pose
This kind of intervention seems fine, though pretty minor from my perspective. I doubt that this will be all that important for all that long.
2. Higher priorities for governments
The whole idea of asking companies to write up AI Safety Policies strikes me as useful, but much less important than some other steps governments should take to address existential risk from smarter-than-human AI. Off the top of my head, governments should also:
set compute thresholds for labs, and more generally set capabilities thresholds; centralize and monitor chips; indefinitely halt the development of improved chips; set up independent risk assessments; and have a well-developed plan for what we’re supposed to do when we get to the brink.Saying a few more words about #4 (which I haven’t seen others discussing much):
I recommend setting up some sort of panel of independent actuaries who assess the risks coming from major labs (as distinct from the value on offer), especially if those actuaries are up to the task of appreciating the existential risks of AGI, as well as the large-scale stakes (all of the resources in the reachable universe, and the long-term role of humanity in the cosmos) involved.
Independent risk assessments are a key component in figuring out whether labs should be allowed to continue at all. (Or, more generally and theoretically-purely, what their “insurance premiums” should be, with the premiums paid immediately to the citizens of earth that they put at risk, in exchange for the risk.4)
Stepping back a bit: What matters is not what written-up answers companies provide to governments about their security policies or the expertise of their red teams; what matters is their actual behavior on the ground and the consequences that result. There’s an urgent need for mechanisms that will create consensus estimates of how risky companies actually are, so that we don’t have to just take their word for it when every company chimes in with “of course we’re being sufficiently careful!”. Panels of independent actuaries who assess the risks coming from major labs are a way of achieving that.
Saying a few more words about #5 (which I also haven’t seen others discussing much): suppose that the evals start triggering and the labs start saying “we cannot proceed safely from here”, and we find that small research groups are not too far behind the labs: what then? It’s all well and good to hope that the issues labs run into will be easy to resolve, but that’s not my guess at what will happen.
What is the plan for the case where the result of “identifying and addressing emerging risks” is that we identify a lot of emerging risks, and cannot address them until long after the technology is widely and cheaply available? If we’re taking those risks seriously, we need to plan for those cases now.
You might think I’d put technical AI alignment research (outside of labs) as another priority on my list above. I haven’t, because I doubt that the relevant actors will be able to evaluate alignment progress. This poses a major roadblock both for companies and for regulators.
What I’d recommend instead is investment in alternative routes (whole-brain emulation, cognitive augmentation, etc.), on the part of the research community and governments. I would also possibly recommend requiring relatively onerous demonstrations of comprehension of model workings before scaling, though this seems difficult enough for a regulatory body to execute that I mostly think it’s not worth pursuing. The important thing is to achieve an indefinite pause on progress toward smarter-than-human AI (so we can potentially pursue alternatives like WBE, or buy time for some other miracle to occur); if “require relatively onerous demonstrations of comprehension” interferes at all with our ability to fully halt progress, and to stay halted for a very long time, then it’s probably not worth it.
If the UK government is maintaining a list of interventions like this (beyond just politely asking labs to be responsible in various ways), I haven’t seen it. I think that eliciting AI Safety Policies from companies is a fine step to be taking, but I don’t think it should be the top priority.
3. Thoughts on the submitted AI Safety Policies
Looking briefly at the individual companies’ stated policies (and filling in some of the gaps with what I know of the organizations), I’d say on a skim that none of the AI Safety Policies meet a “basic sanity / minimal adequacy” threshold — they all imply imposing huge and unnecessary risks on civilization writ large.
In relative terms:
The best of the policies seems to me to be Anthropic’s, followed closely by OpenAI’s. I lean toward Anthropic’s being better than OpenAI’s mainly because Anthropic’s RSP seemed to take ASL-4 more seriously as a possibility, and give it more lip service. But it’s possible that I just missed some degree of seriousness in OpenAI’s RDP for one reason or another. DeepMind’s policy seemed a lot worse to me, followed closely by Microsoft’s. Amazon’s policy struck me as far worse than Microsoft’s. Meta had the worst stated policy, far worse than Amazon’s.Anthropic and OpenAI pass a (far lower, but still relevant) bar of “making lip service to many of the right high-level ideals and priorities”. Microsoft comes close to that bar, or possibly narrowly meets it (perhaps because of its close relationship to OpenAI). DeepMind’s AI Safety Policy doesn’t meet this bar from my perspective, and lands squarely in “low-content corporate platitudes” territory.
Matthew Gray read the policies more closely than me (and I respect his reasoning on the issue), and writes:
Unlike Nate, I’d rank Anthropic and OpenAI’s write-ups as ~equally good. Mostly I think comparing their plans will depend on how OpenAI’s Risk-Informed Development Policy compares to Anthropic’s Responsible Scaling Policy.5 For now, only Anthropic’s RSP has shipped, and we’re waiting on OpenAI’s RDP.
I’d also rank DeepMind’s write-up as far better than Microsoft’s, and Amazon’s as only modestly worse than Microsoft’s. Otherwise, I agree with Nate’s rankings.
Soares: Anthropic > OpenAI >> DeepMind > Microsoft >> Amazon >> Meta Gray: OpenAI ? Anthropic >> DeepMind >> Microsoft > Amazon >> MetaBy comparison, CFI’s recent rankings look like the following (though CFI’s reviewers were only asking whether these companies’ AI Safety Policies satisfy the UK government’s requirements, not asking whether these policies are good):6
CFI reviewers: Anthropic >> DeepMind ? Microsoft ? OpenAI >> Amazon >> MetaMy read of the policy write-ups was:
Anthropic | Believes in evals and responsible scaling; aspirational about security. (In contrast to more established tech companies, which can point to their cybersecurity expertise over decades, Anthropic’s proposal can only point to them advocating for strengthening cybersecurity controls at frontier AI labs.) |
OpenAI | Believes in alignment research; decent on security. I think OpenAI’s “we’ll solve superalignment in 4 years!” plan is wildly unrealistic, but I greatly appreciate that they’re acknowledging the problem and sticking their neck out with a prediction of what’s required to solve it; I’d like to see more falsifiable plans from other organizations about how they plan to address alignment. |
DeepMind | Believes in scientific progress; takes security seriously. |
Microsoft | Experienced tech company, along for the ride with OpenAI. My read is that Microsoft is focused on the short-term security risks; they seem to want to operate a frontier AI model datacenter more than they want to unlock an intelligence explosion. |
Amazon | Experienced tech company; wants to sell products to customers; takes security seriously. Like DeepMind, Amazon provides detailed answers on the security questions that point to lots of things they’ve done in the past.7 |
Meta | Believes in open source; fighting the brief at several points. |
I see these policies as largely discussing two different things: security (e.g., making it difficult for outside actors to steal data from your servers, like your model weights), and safe deployment (not accidentally releasing something that goes rogue, not enabling a bad customer to do something catastrophic, etc.).
These involve different skill sets, and different sets of companies seem to me to be most credible on one versus the other. E.g,, Anthropic and OpenAI seemed to be thinking the most seriously about safe deployment, but I don’t think Anthropic or OpenAI have security as impressive as Amazon-the-company (though I don’t know about the security standards of Amazon’s AI development teams specifically).
In an earlier draft, Nate ranked Microsoft’s response as better than DeepMind’s, because “DeepMind seemed more like it was trying to ground things out into short-term bias concerns, and Microsoft seemed on a skim to be throwing up less smoke and mirrors.” However, I think DeepMind’s responses were equal or better than Microsoft’s on all nine categories.
On my read, DeepMind’s answers contained a lot of “we’re part of Google, a company that has lots of experience handling this stuff, you can trust us”, and these sections brought up near-term bias issues as part of Google’s track record. However, I think this wasn’t done in order to deflect or minimize the problem. E.g., DeepMind writes:
This could include cases where the AI system exhibits misaligned or biassed behaviour; the AI system assists the user to perform a highly dangerous task (e.g. assemble a bioweapon); new jailbreak prompts; or security vulnerabilities that undermine user data privacy.
The first part of this quote looks like it might be a deflection, but DeepMind then explicitly flags that they have catastrophic risks in mind (“assemble a bioweapon”). In contrast, Microsoft never once brings up biorisks, catastrophes, nuclear risks, etc. I think Microsoft wants to make sure their servers aren’t hacked and they comply with laws, whereas DeepMind is thinking about the fact that they’ve made something you could use to help you kill someone.
The part of DeepMind’s response that struck me as smoke-and-mirrors-ish was instead their choice to redirect a lot of the conversation to abstract discussions of scientific and technological progress. For example, while talking about monitoring AlphaFold usage, they talk about using logs of usage to tally the benefits to the research community instead of any actual “monitoring” benefit, like whether or not users were generating proteins that could be useful for harming others. While it is appropriate to weigh both the benefits and the risks of new technology, subtly changing the subject from how risks are being monitored to benefits seems like a distraction.
My argument here was enough to persuade Nate on this point, and he updated his ranking to place DeepMind a bit higher than Microsoft.
I think evaluating the proposals has the downside that, unless someone fights the brief or gives a concretely dumb answer somewhere, there isn’t actually much to evaluate. One company offers $15k maximum for a bug bounty, another company $20k maximum; does that matter? Did another company which didn’t write a number offer more, or less?
Meaningfully evaluating these companies will likely require looking at the companies’ track records and other statements (as Nate and I both tried to do to some degree), rather than looking at these policies in isolation. These considerations also make me very interested in Nate’s proposal of using independent actuaries to assess labs’ risk.
Inflection is a late addition to the list, so Matt and I won’t be reviewing their AI Safety Policy here.
Thanks to Rob Bensinger for helping heavily edit and revise this post. ?

“Our current techniques for aligning AI, such as reinforcement learning from human feedback, rely on human ability to supervise AI. But these techniques will not work for superintelligence, because humans will be unable to reliably supervise AI systems much smarter than us.” ?



“This is why we are building on our industry-leading general and infrastructure security approach. Our models are developed, trained, and stored within Google’s infrastructure, supported by central security teams and by a security, safety and reliability organisation consisting of engineers and researchers with world-class expertise. We were the first to introduce zero-trust architecture and software security best practices like fuzzing at scale, and we have built global processes, controls, and systems to ensure that all development (including AI/ML) has the strongest security and privacy guarantees. Our Detection & Response team provides a follow-the-sun model for 24/7/365 monitoring of all Google products, services and infrastructure – with a dedicated team for insider threat and abuse. We also have several red teams that conduct assessments of our products, services, and infrastructure for safety, security, and privacy failures.” ?
The post Thoughts on the AI Safety Summit company policy requests and responses appeared first on Machine Intelligence Research Institute.