By John P. Desmond, AI Trends Editor The AI stack defined by Carnegie Mellon University is fundamental to the approach being taken by the US Army for its AI development platform efforts, according to Isaac Faber, Chief Data Scientist...
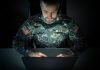
By John P. Desmond, AI Trends Editor
The AI stack defined by Carnegie Mellon University is fundamental to the approach being taken by the US Army for its AI development platform efforts, according to Isaac Faber, Chief Data Scientist at the US Army AI Integration Center, speaking at the AI World Government event held in-person and virtually from Alexandria, Va., last week.
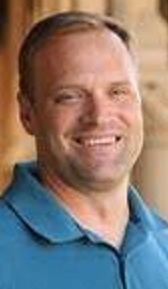
“If we want to move the Army from legacy systems through digital modernization, one of the biggest issues I have found is the difficulty in abstracting away the differences in applications,” he said. “The most important part of digital transformation is the middle layer, the platform that makes it easier to be on the cloud or on a local computer.” The desire is to be able to move your software platform to another platform, with the same ease with which a new smartphone carries over the user’s contacts and histories.
Ethics cuts across all layers of the AI application stack, which positions the planning stage at the top, followed by decision support, modeling, machine learning, massive data management and the device layer or platform at the bottom.
“I am advocating that we think of the stack as a core infrastructure and a way for applications to be deployed and not to be siloed in our approach,” he said. “We need to create a development environment for a globally-distributed workforce.”
The Army has been working on a Common Operating Environment Software (Coes) platform, first announced in 2017, a design for DOD work that is scalable, agile, modular, portable and open. “It is suitable for a broad range of AI projects,” Faber said. For executing the effort, “The devil is in the details,” he said.
The Army is working with CMU and private companies on a prototype platform, including with Visimo of Coraopolis, Pa., which offers AI development services. Faber said he prefers to collaborate and coordinate with private industry rather than buying products off the shelf. “The problem with that is, you are stuck with the value you are being provided by that one vendor, which is usually not designed for the challenges of DOD networks,” he said.
Army Trains a Range of Tech Teams in AI
The Army engages in AI workforce development efforts for several teams, including: leadership, professionals with graduate degrees; technical staff, which is put through training to get certified; and AI users.
Tech teams in the Army have different areas of focus include: general purpose software development, operational data science, deployment which includes analytics, and a machine learning operations team, such as a large team required to build a computer vision system. “As folks come through the workforce, they need a place to collaborate, build and share,” Faber said.
Types of projects include diagnostic, which might be combining streams of historical data, predictive and prescriptive, which recommends a course of action based on a prediction. “At the far end is AI; you don’t start with that,” said Faber. The developer has to solve three problems: data engineering, the AI development platform, which he called “the green bubble,” and the deployment platform, which he called “the red bubble.”
“These are mutually exclusive and all interconnected. Those teams of different people need to programmatically coordinate. Usually a good project team will have people from each of those bubble areas,” he said. “If you have not done this yet, do not try to solve the green bubble problem. It makes no sense to pursue AI until you have an operational need.”
Asked by a participant which group is the most difficult to reach and train, Faber said without hesitation, “The hardest to reach are the executives. They need to learn what the value is to be provided by the AI ecosystem. The biggest challenge is how to communicate that value,” he said.
Panel Discusses AI Use Cases with the Most Potential
In a panel on Foundations of Emerging AI, moderator Curt Savoie, program director, Global Smart Cities Strategies for IDC, the market research firm, asked what emerging AI use case has the most potential.
Jean-Charles Lede, autonomy tech advisor for the US Air Force, Office of Scientific Research, said,” I would point to decision advantages at the edge, supporting pilots and operators, and decisions at the back, for mission and resource planning.”
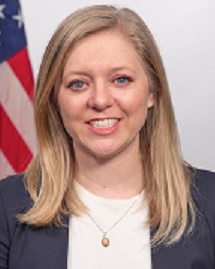
Krista Kinnard, Chief of Emerging Technology for the Department of Labor, said, “Natural language processing is an opportunity to open the doors to AI in the Department of Labor,” she said. “Ultimately, we are dealing with data on people, programs, and organizations.”
Savoie asked what are the big risks and dangers the panelists see when implementing AI.
Anil Chaudhry, Director of Federal AI Implementations for the General Services Administration (GSA), said in a typical IT organization using traditional software development, the impact of a decision by a developer only goes so far. With AI, “You have to consider the impact on a whole class of people, constituents, and stakeholders. With a simple change in algorithms, you could be delaying benefits to millions of people or making incorrect inferences at scale. That’s the most important risk,” he said.
He said he asks his contract partners to have “humans in the loop and humans on the loop.”
Kinnard seconded this, saying, “We have no intention of removing humans from the loop. It’s really about empowering people to make better decisions.”
She emphasized the importance of monitoring the AI models after they are deployed. “Models can drift as the data underlying the changes,” she said. “So you need a level of critical thinking to not only do the task, but to assess whether what the AI model is doing is acceptable.”
She added, “We have built out use cases and partnerships across the government to make sure we’re implementing responsible AI. We will never replace people with algorithms.”
Lede of the Air Force said, “We often have use cases where the data does not exist. We cannot explore 50 years of war data, so we use simulation. The risk is in teaching an algorithm that you have a ‘simulation to real gap’ that is a real risk. You are not sure how the algorithms will map to the real world.”
Chaudhry emphasized the importance of a testing strategy for AI systems. He warned of developers “who get enamored with a tool and forget the purpose of the exercise.” He recommended the development manager design in independent verification and validation strategy. “Your testing, that is where you have to focus your energy as a leader. The leader needs an idea in mind, before committing resources, on how they will justify whether the investment was a success.”
Lede of the Air Force talked about the importance of explainability. “I am a technologist. I don’t do laws. The ability for the AI function to explain in a way a human can interact with, is important. The AI is a partner that we have a dialogue with, instead of the AI coming up with a conclusion that we have no way of verifying,” he said.
Learn more at AI World Government.