In its latest report on implementing Generative AI (Gen AI) in the banking industry, The McKinsey Global Institute’s estimations underscore the staggering potential this technology holds, projecting an annual value addition of US$2.6 trillion to US$4.4 trillion across various...
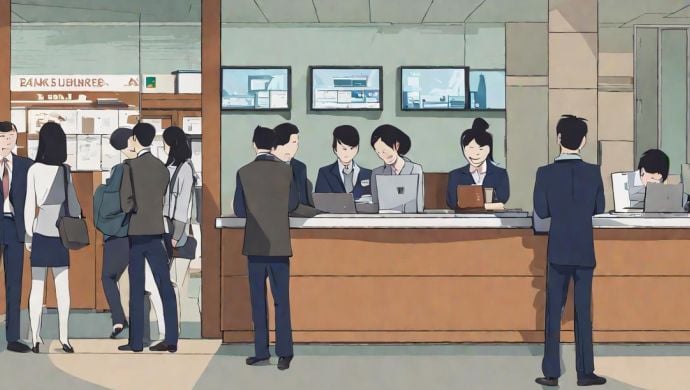
In its latest report on implementing Generative AI (Gen AI) in the banking industry, The McKinsey Global Institute’s estimations underscore the staggering potential this technology holds, projecting an annual value addition of US$2.6 trillion to US$4.4 trillion across various sectors globally. Among these, the banking sector stands out with a potential annual windfall of US$200 billion to US$340 billion, equivalent to nine to 15 per cent of operating profits, primarily attributed to heightened productivity.
However, the journey towards harnessing the full potential of Gen AI is not without its unique challenges.
“For banks seeking to tap this valuable technology, a Gen AI scale-up is in some ways like any other—it requires old-school change management skills, upfront senior leadership alignment and sponsorship, business-unit accountability for results, value-centred use cases, clear targets, and so on. In other ways, a Gen AI scale-up is like nothing most leaders have ever seen,” the report stated.
Firstly, the sheer scope of the task is monumental, necessitating a comprehensive understanding of intricate AI concepts. The sudden immersion of banking leaders into the world of reinforcement learning and convolutional neural networks reflects the urgency to adapt strategically. Management teams must navigate through potential pathways and position themselves strategically to harness the diverse capabilities of this transformative technology.
Secondly, the integration of Gen AI introduces a complexity that disrupts the established balance between business and technology within financial institutions. While advancements such as agile methodologies and cloud integration addressed the historical divide, the prominence of analytics and data as a critical coordination node complicates the operating dynamic. Gen AI demands more profound data and analytics integration throughout the value chain, requiring business leaders to collaborate more closely with analytics experts.
Also Read: Navigating the AI landscape in 2024: Why there is an urgency for enhanced governance
The unprecedented pace of change is the third factor accelerating the urgency of Gen AI adoption. Unlike the gradual shift towards mobile banking, Gen AI tools are swiftly becoming integral to banking operations. The use of AI-based tools by financial giants such as Goldman Sachs to automate labour-intensive processes exemplifies the rapid assimilation into everyday practices. For slower-moving organisations, this accelerated change can strain existing operating models.
Lastly, the talent-related challenges associated with scaling up Gen AI cannot be overstated. Leading banks with established teams of AI experts may have a head start, but others need to bridge the gap through a combination of training and recruitment. The demand for skills such as prompt engineering and database curation necessitates a strategic approach to talent acquisition.
A successful transformation
The report suggests seven steps that the banking industry can take to implement digital transformation with Gen AI successfully:
Strategic Roadmap
Management teams should develop a comprehensive strategic view of where Gen AI and advanced analytics fit into their business. This roadmap should encompass transformative business model changes and tactical improvements, allowing leaders to make adaptive decisions on investment and implementation.
Talent Acquisition
Leaders must personally understand gen AI and invest in executive education to bridge the knowledge gap within their teams. This approach generates excitement and addresses concerns among employees, ensuring a smoother transition.
Also Read: Unlock growth potential with the latest insights on Gen-AI
Operating Model
Rather than a new “Gen AI operating model,” successful institutions should adapt their existing models for flexibility and scalability. Cross-functional teams that align accountabilities and responsibilities between delivery and business teams are crucial for coherence and transparency.
Technology Choices
Carefully considering whether to build, buy, or partner is vital for successful Gen AI integration. Decisions on foundational models, cloud infrastructure, and MLOps platforms should align with the bank’s overall strategy.
Data Management
Given Gen AI’s reliance on unstructured data, banks must reassess their data strategies and architectures. The ability to leverage unstructured data facilitated by Gen AI is a key consideration.
Risk and Controls
With the boost in productivity, Gen AI introduces new risks, necessitating a redesign of risk- and model-governance frameworks. Banks must proactively develop controls to mitigate potential challenges.
Adoption and Change Management
A well-thought-out application can stall without effective change management. Encouraging employees and customers to embrace Gen AI requires careful design, addressing comfort levels and ensuring clear executive support.
As the banking industry embarks on the journey of scaling Gen AI, the successful navigation of these seven points will be pivotal in unlocking the full potential of this transformative technology. While challenges abound, the promise of enhanced productivity and profitability propels the industry towards a future where gen AI becomes an integral force in shaping banking operations.
—
Image Credit: RunwayML
The article was first published on December 6, 2023.
The post Gen AI in banking: How to ensure a successful transformation for an age-old industry appeared first on e27.